Ghost Work - The Invisible Back-End of AI
- debdut pramanick
- Mar 17
- 4 min read
The advent of artificial intelligence has dazzled the world, showing us the glittering heights to which technology can soar. From self-driving cars to chatbots that mimic human conversation, AI has reshaped industries, promising efficiency, intelligence and automation on an unprecedented scale. But as history has often warned us, all that glitters has an unseen and darker side.
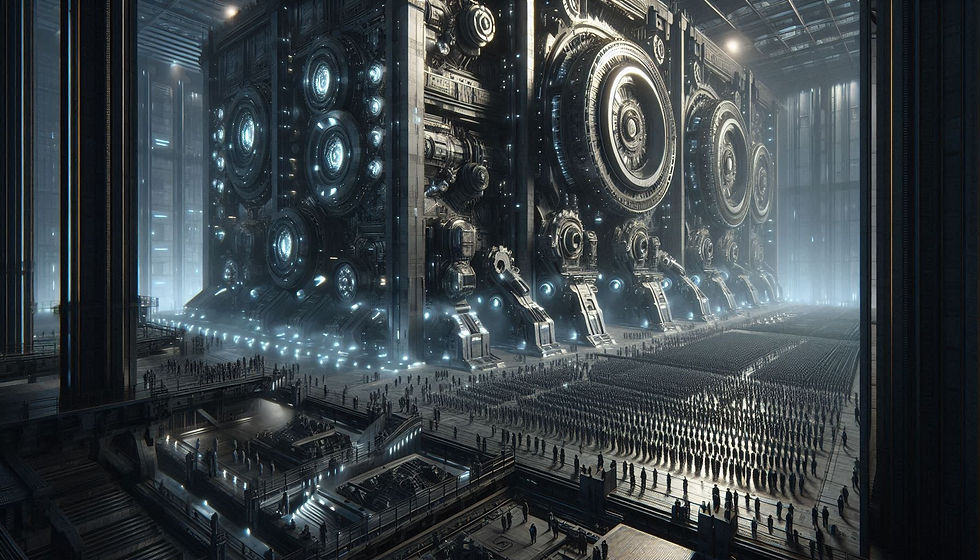
Behind the seamless interfaces and polished algorithms of AI lies an unsettling reality—one reminiscent of the dystopian sci-fi worlds we’ve seen in movies. In these cautionary tales, we see towering machines, gleaming in their technological supremacy, served by thousands of nameless, faceless human laborers toiling in the shadows. These workers, unseen and unrecognized, ensure that the machines function flawlessly, yet they themselves remain expendable—forgotten gears in a system that thrives on their silent effort.
AI is no different. Beneath its sleek veneer exists a hidden workforce: ghost workers who painstakingly train the algorithms that power the Large Language Models. Their work is essential, yet it is invisible. Their labor fuels progress, yet they receive neither recognition nor fair compensation.
In the world of artificial intelligence (AI), the term "ghost work" has emerged to describe a crucial yet often overlooked segment of the workforce responsible for data annotation. This invisible back-end labor is essential for training machine learning models, yet it remains largely unrecognized and undervalued.
As we marvel at the wonders of AI, it’s time we confront the uncomfortable truth—that these unseen human hands are the real foundation of the technology we so readily embrace.
This is the story of ghost work—the hidden back-end of AI.
Understanding Data Annotation
At its core, data annotation involves labeling and tagging various forms of content—such as text, images, audio, and video—so that AI and machine learning models can recognize and interpret them.
This process is fundamental to transforming raw data into structured datasets that machines can understand. For instance, in image recognition tasks, annotators might label objects within images to help algorithms learn to identify those objects in new, unseen images.
The importance of data annotation cannot be overstated; it serves as the foundation for machine learning models to learn patterns, make decisions, and perform tasks accurately. Without high-quality annotated data, AI systems would struggle to function effectively. According to estimates from NASSCOM, up to 1 million Indians could be engaged in full-time or part-time data annotation by 2030, reflecting the growing demand for this type of work.
The Nature of Manual Annotation Work
Manual data annotation is labor-intensive and often repetitive. Annotators may be tasked with a variety of jobs, including:
Image Labeling: Identifying objects within images and marking them accordingly.
Text Annotation: Tagging parts of speech in sentences or identifying sentiment in text.
Audio Transcription: Converting spoken language into written text.
Video Annotation: Labeling actions or objects within video frames.
Many individuals engaged in this work are freelancers or gig workers who operate under precarious conditions. Reports suggest that approximately 50,000 freelancers work as ghost workers, often earning just a few cents per task. These workers typically face uncertain hours and wages, making their financial stability precarious. The working conditions for data annotators can be challenging. Many operate in environments that prioritize productivity over well-being, leading to mental fatigue and burnout. Long hours spent performing monotonous tasks can take a toll on physical and mental health. Furthermore, many annotators lack job security and benefits typically associated with full-time employment, such as health insurance or paid leave.
The Business Model Behind Ghost Work
The demand for data annotation services is driven by companies eager to leverage AI technologies for competitive advantage. Businesses purchase these services from call centers or specialized firms that employ ghost workers under low-cost labor models. Companies are attracted to this arrangement for several reasons:
Cost-Effectiveness: Data annotation is often seen as a low-skill job that can be outsourced at minimal expense. This allows companies to save on labor costs while still obtaining the necessary annotated data.
Scalability: As AI projects grow in complexity and scale, the need for large volumes of annotated data increases. Outsourcing this work enables companies to quickly ramp up their data labeling efforts without investing heavily in infrastructure or full-time employees.
Access to a Global Workforce: Many companies source their data annotation needs from countries like India, where labor costs are lower. This global approach allows businesses to tap into a vast pool of potential workers who can provide the required services at competitive rates.
However, this model raises significant ethical concerns regarding worker treatment and compensation. Ghost workers often find themselves at the bottom of the AI value chain with little opportunity for advancement or wage increases.
The Current Landscape of Ghost Work
The phenomenon of ghost work highlights a critical aspect of the AI ecosystem that is frequently ignored—the human effort behind machine learning advancements.
As AI continues to evolve and permeate various sectors, the reliance on data annotation will only grow. While there is a strong policy push for India to become a global hub for AI production, it is essential that this growth does not come at the expense of worker welfare.
Currently, many ghost workers endure low wages and poor working conditions while contributing significantly to the development of AI technologies. As we move forward into an increasingly automated future, it is imperative that stakeholders—including governments, companies, and consumers—recognize the value of these workers and advocate for fair labor practices.
References:
Comments